A Comprehensive Review and Comparative Evaluation of Pedestrian Crash Prediction Approaches
Synopsis
Road crashes represent a significant public health concern, causing immense human suffering and economic losses worldwide. Vulnerable road users, such as pedestrians, cyclists, three-wheelers and motorcyclists, account for half of all deaths on roadways. Among them, pedestrians alone contribute to more than half of all fatalities, highlighting the severity of the dangers they face. Crash prediction studies play an important role in identifying high-risk areas and implementing measures to mitigate pedestrian crashes, thus actively contributing to the reduction of pedestrian fatalities and injuries on roadways. Through a comprehensive literature review, it was found that during the 1960s, pedestrian safety studies gained prominence, indicating a significant shift in recognizing the importance of pedestrian safety. Statistical models such as Multiple Linear Regression, Poisson Regression, and Negative Binomial Regression were introduced to analyze the relationship between pedestrian crashes and various factors, including traffic volume, road characteristics, and environmental variables. Moreover, recent advancements in machine learning have enhanced the accuracy and predictive capabilities of pedestrian crash prediction models. The present review paper explains a comparative analysis between statistical modeling and machine learning modeling as applied to crash prediction modeling. Machine learning models, adept at handling large and complex datasets, generally offer higher predictive accuracy compared to statistical models, which are more suitable for smaller datasets and understanding causal relationships. In both statistical modeling and machine learning approaches, common parameters such as traffic volume, road characteristics, and environmental variables are utilized for pedestrian crash prediction, while the consideration of pedestrian behaviour factors remains limited.
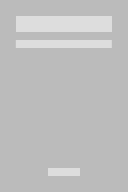

This work is licensed under a Creative Commons Attribution-NonCommercial 4.0 International License.