MACHINE LEARNING CLASSIFICATION MODELS FOR SEISMIC VULNERABILITY OF BUILDINGS
Synopsis
Predicting structural performance under seismic excitation and damage after the seismic event plays a vital role in life safety and economics. This study examines the vulnerability to earthquakes of a two-story 2D steel moment-resisting frame (SMRF) frame using the OpenSeesPy framework. The study utilizes non-linear static and time history analyses to evaluate the structures' reaction to seismic forces. The pushover analysis offers vital insights into the nonlinear behaviour of structures, whereas time history analysis considers the actual ground motion patterns in real-time. There is a lack of comprehensive assessments of machine learning (ML) progress within different areas of structural engineering. This includes a thorough examination of existing literature that can offer a timely evaluation of methods for assessing risk and resilience in the built environment. This study examines the performance of the selected ML classification techniques, such as Random Forest(RF), Logistic Regression, Decision Tree, K-Nearest Neighbours(KNN), LightGBM, CatBoost, Naïve Bayes, XGBoost, and AdaBoost. Binary classification is done to classify the data set as having low and high inter-storey drift. These predictions are useful to the government and other private companies for preparing an effective methodology to be followed after seismic events, efficient retrofitting and rehabilitation to extend the durability of existing structures, insurance estimates, decision-making in disaster risk reduction, and others.
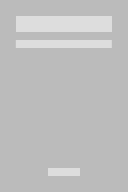

This work is licensed under a Creative Commons Attribution-NonCommercial 4.0 International License.