Evaluation of LLMs Capacity to Assist in Chemistry Laboratory Courses
Synopsis
Large language models (LLMs) have emerged as exceptional assistants across many domains of education. However, their efficacy in assisting with chemistry laboratory courses still needs to be explored. The present study will evaluate the capacities of several LLMs, including Claude, Gemini, ChatGPT, and others, to perform tasks relevant to chemistry education through a series of queries. The result demonstrated LLMs excel at retrieving complex information, generating well-structured experimental manuals, clarifying chemical concepts with exclusive insights, and predicting reactive sites of a given compound. However, LLMs still needed to process the chemical structure representation more successfully and identify compounds by their IUPAC names. The challenge in these domains emerge from LLMs' query reformulation and the rendering of partial response methods used to produce a response to any question. To overcome these challenges, the present study suggests integrating LLMs with cheminformatics toolkits like RDKit and providing access to standard chemical databases. By strategically combining LLMs' natural language capabilities with specialized chemical data processing functionalities, the role of LLMs in enhancing chemistry learning experiences can be fully harnessed. Therefore, the demonstrations of the present study will lay the groundwork for the future development of more effective AI-powered education tools.
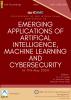

This work is licensed under a Creative Commons Attribution-NonCommercial 4.0 International License.