Leveraging Machine Learning for the Management of Ureteropelvic Confluence Obstruction
Synopsis
Prompt detection of Ureteropelvic Junction obstruction is a vital precursor to renal impairment and is indispensable for early treatment. This paper presents a novel approach that combines real-time ultrasound imagery with advanced Machine Learning techniques. This method begins with data collection, as in this paper record real time ultrasound images and carefully preprocess and standardize these images to ensure consistency and reliability. At the heart of the methodology is feature extraction, as this paper utilizes classification yielded by a pre trained MobileNetV2 model to extract informative feature vectors from the ultrasound images. These vectors are then used to dictate the presence or absence of UPJ obstruction, allowing to train a Support Vector Machine model. The SVM model is good at differentiating fresh ultrasound images and predicting whether they are indicative of UPJ obstruction or normal conditions, providing a valuable socio economic diagnostic resource. The model evaluation involved the partitioning of the dataset into training and testing subsets, using accuracy metrics as benchmarks of performance assessment. Additionally, visualization techniques including confusion matrices and T Distributed Stochastic Neighbour Embedding were utilized to strengthen result interpretability. The model in this paper shows great potential as a non-invasive, cost-effective tool for the detection of UPJ obstruction. Ultrasound imaging and machine learning seek to revolutionize the field of early diagnosis and intervention of this pathology. This will ultimately lead to better patient outcomes.
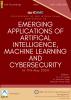

This work is licensed under a Creative Commons Attribution-NonCommercial 4.0 International License.