Identification of Exoplanets using Machine Learning
Synopsis
Over the past decade more than a million stars have been observed as part of efforts to identify transiting planets. Identifying potential exoplanet candidates requires manual interpretation which is both labor intensive and susceptible to human error the consequences of which are often difficult to quantify. This paper describes a novel approach that utilizes a neural network to automate the identification of potential exoplanet candidates in large planetary search programs a method that contrasts with traditional manual techniques. A combination of classification models and diverse datasets are employed to assess the probability that an object is an exoplanet. The first step of the process involves cleaning raw astronomical images obtained from reliable sources removing extraneous objects and disturbances introduced by noise and electromagnetic radiation. Once the data is cleaned categorization models are applied to analyze and classify the objects. This approach significantly reduces the need for manual intervention and enhances the accuracy and efficiency of exoplanet detection. By automating the detection process it enables more extensive planetary search programs to operate more effectively thus contributing to the advancement of exoplanet research. This paper offers a more reliable and scalable solution to the identification of exoplanet candidates.
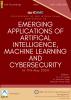

This work is licensed under a Creative Commons Attribution-NonCommercial 4.0 International License.