Machine Learning Approaches for Predicting Mechanical Properties of Composite Materials
Synopsis
Due to their lightweight and superior mechanical properties, composite materials are gaining in popularity. It is important to predict these properties for the design and optimization of composite materials. However, traditional experimentation methods are time-consuming. The use of machine learning methods to predict the mechanical properties in composite materials has become a promising alternative. The purpose of this research is to compare and explore different machine-learning approaches including Artificial Neural Networks (ANNs), Support Vector Regression (SVR), Random Forests (RF), Gradient Boosting Machines (GBM), as well as deep learning techniques like convolutional and recurrent networks. Data collection, feature selection and model training are all part of the methodology. Each algorithm's performance will be measured using the appropriate metrics and then, the best algorithms will be selected. Discussions will include the practical implications, advantages, limitations and future directions of research. The study provides insights on the performance and applicability of machine-learning techniques to predict the mechanical properties in composite materials. These results have the potential of accelerating the development and adoption of composite materials with high performance in different industries.
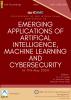

This work is licensed under a Creative Commons Attribution-NonCommercial 4.0 International License.