Enhancing and Optimizing Image Quality Assessment with CNNs and AI Techniques
Synopsis
This paper presents a comprehensive exploration of optimizing Image Quality Assessment (IQA) for image processing systems through the integration of Convolutional Neural Networks (CNNs) and advanced AI techniques. The study delves into the multifaceted approach of leveraging CNNs to enhance IQA performance, focusing on critical aspects such as data preprocessing, model architecture selection, and model fusion methodologies. The framework proposed in this paper aims to revolutionize image processing applications by significantly improving IQA accuracy and robustness. By addressing key challenges in IQA, such as handling diverse image distortions and improving perceptual quality estimation, this framework has the potential to advance the state-of-the-art in image quality evaluation. Moreover, the paper highlights the broader implications of enhancing IQA accuracy and robustness, emphasizing the transformative impact it could have on various fields, including medical imaging, autonomous vehicles, and multimedia communication. The integration of advanced AI techniques and CNNs in IQA optimization is expected to not only enhance the quality of image processing systems but also pave the way for innovative applications in the future.
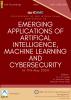

This work is licensed under a Creative Commons Attribution-NonCommercial 4.0 International License.