Crop Production Prediction Using Ensemble Neural Network Model
Synopsis
This study presents a pioneering methodology for predicting and classifying crop production, leveraging an Ensembled Neural Network Model (ENNM) that integrates the Squeeze Net and Efficient Net architectures. By amalgamating the strengths of these models, our approach aims to significantly enhance the accuracy and efficiency of crop production forecasting. Squeeze Net’s lightweight design and Efficient Net’s superior performance collectively contribute to a robust framework capable of tackling the complexities of agricultural data. Through extensive experimentation on a diverse crop production dataset, we demonstrate the efficacy of our proposed ENNM, achieving an outstanding accuracy rate of 98.5%. It enhances the model's adaptability to diverse agricultural environments and cropping systems. Our findings signify a significant step forward in harnessing advanced machine learning (ML) techniques for addressing real-world challenges in agriculture, with implications for improving crop yield forecasts, optimizing resource allocation, and bolstering food security efforts on a global scale.
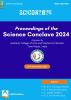

This work is licensed under a Creative Commons Attribution-NonCommercial 4.0 International License.