Machine Learning Approaches for Predicting Autism in Children: A Comparison of AdaBoost and Other Algorithms
Synopsis
This study presents a Autism Spectrum Disorder (ASD) in children is a neurodevelopmental disorder characterized by challenges in social interactions, communication, and behavior. Early identification and diagnosis of ASD, especially between the ages of 20 and 60 months, are essential for effective treatment. Without early detection, intervention becomes significantly more difficult. While a variety of machine learning (ML) techniques have been employed to predict ASD, the accuracy of predictions for younger age groups is still limited. This study investigates the use of three machine learning algorithms—Support Vector Machine (SVM), Random Forest, and AdaBoost—to predict and identify autism in children. The AdaBoost classifier, which integrates multiple weak learners to form a more robust classifier, is proposed as the primary approach. To assess the performance of these algorithms, key metrics such as accuracy, precision, F-score, and the confusion matrix are computed. The algorithm with the highest accuracy is then utilized to forecast autism in children.
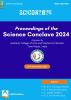

This work is licensed under a Creative Commons Attribution-NonCommercial 4.0 International License.