Advanced Machine Learning Techniques for Intrusion Detection System Development
Synopsis
Intrusion Detection Systems (IDS) play a critical role in safeguarding digital infrastructures against evolving cyber threats. This research explores the integration of advanced machine learning (ML) techniques to enhance the detection accuracy and adaptability of IDS. Traditional IDS methodologies often struggle with scalability, false positives, and identifying novel attack patterns. To address these challenges, this study leverages advanced ML algorithms, including deep learning architectures, ensemble models, and anomaly detection techniques, for effective threat identification. A comprehensive dataset is utilized to evaluate system performance, ensuring robust benchmarking across various metrics such as accuracy, precision, recall, and F1-score. Furthermore, feature selection and dimensionality reduction methods are employed to optimize computational efficiency while maintaining predictive performance. The results demonstrate that advanced ML techniques significantly improve intrusion detection capabilities compared to conventional methods, particularly in detecting zero-day attacks and minimizing false positives. This paper concludes with a discussion on the implications of integrating advanced ML models into real-world IDS applications and outlines future directions for research in this domain.
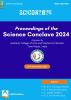

This work is licensed under a Creative Commons Attribution-NonCommercial 4.0 International License.