A Rule-Based Framework for Predicting Rainfall Using Decision Trees and Fuzzy Petri Nets
Synopsis
In a crucial component of meteorology is weather prediction, and precise forecasting has a big impact on everyday life, agriculture, and disaster relief. Based on past meteorological data, this study investigates the possibility of data mining approaches for forecasting rainfall and other weather conditions. We apply the C4.5 decision tree algorithm to a dataset of 2,500 weather observations in order to categorize meteorological conditions and find trends that affect the likelihood of rainfall. We use fuzzy Petri nets to handle the inherent uncertainty in meteorological data, enabling a more adaptable, sophisticated understanding of probabilistic weather patterns. Our combined strategy provides a well-balanced framework that makes use of the C4.5 algorithm's strong classification capabilities while improving the model's dependability and interpretability via fuzzy logic. According to preliminary findings, this hybrid strategy offers a viable way to increase the precision of weather forecasts. By demonstrating how data mining and fuzzy algorithms can efficiently support short-term weather forecasting, this study advances the area of data-driven meteorology.
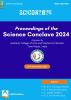

This work is licensed under a Creative Commons Attribution-NonCommercial 4.0 International License.