Application of New Group Contribution Method and Artificial Neural Network-Group Contribution Method in the Prediction of Critical Properties of Pure Hydrocarbons
Synopsis
The aim of this work is to determine the critical properties of pure hydrocarbons by two methods: the method of group contribution with interactions and the method of artificial neural networks. A New group contribution method was developed to predict critical temperatures, pressures and volumes of hydrocarbon compounds by using intramolecular interactions. For neural networks we have developed networks that can approximate the critical properties of pure hydrocarbons which are functions of boiling point and structure of the hydrocarbons. When we compared these methods with other group contributions methods, we have obtained better results and satisfactory accuracy. This study involved two approaches: In the first approach, we proposed new correlations based on the principle of contribution of groups with interactions (IGC) for the prediction of critical properties of six families of pure hydrocarbons: n-paraffins, isoparaffins, olefins, alkynes, naphthenes and aromatics. The deviations obtained by these correlations are relatively small compared to experimental data, and other correlations in the literature. The second approach aimed at predicting critical properties by coupling artificial neural networks to the method of non-interacting group contributions. The established method allows a better prediction of the studied properties compared to conventional methods cited in the literature. The results obtained show small deviations from experimental data including the simulation of neural networks on different isomers. These results justify the addition of the boiling temperature as an additional input next to the compound structure.
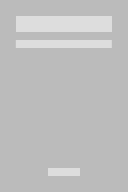

This work is licensed under a Creative Commons Attribution-NonCommercial 4.0 International License.